Proteomics
Proteomics
Proteomics is the global or comprehensive study a proteome, a set of proteins produced by a biological unit such as a cell, tissue, organ, etc. A proteome is dynamic and generally correlates with the transcriptome, the full range of messenger RNA transcribed by a biological unit. However, that correlation may vary, as protein activity and function are modulated by many factors. Proteomics provides a way to investigate when, where, to what extent, and how quickly proteins are expressed and degraded. Many other research questions may also be answered or explored using proteomics.
Extraction and Separation: As has been a feature of protein research for decades, a primary decision in proteomics is protein solubility and preparation of soluble and non-soluble protein fractions. Other factors include source biological material and analytic platform on which samples will be processed. Experience in this phase of the proteomics process is valuable.
Detection: Proteomics relies heavily on mass spectrometry-based techniques with high-throughput capabilities, including time-of-flight and tandem mass spectrometry, as well as gel-based techniques such as differential in-gel electrophoresis, SDS-PAGE, and isoelectric focusing. Nuclear magnetic resonance spectroscopy is also an option.
Untargeted versus Targeted: Untargeted proteomics is generally thought of as a hypothesis-generating, global “look-and-see” analysis. Untargeted proteomics is a powerful discovery platform. Targeted proteomics, an hypothesis-driven approach, becomes the method of choice for validation and quantification of specific proteins or protein pathways.
Informatics: Proteomics datasets are large, and feature-finding can be difficult with only classical statistics. Machine learning approaches increase speed and ease of feature-finding. Unsupervised techniques such as principal components analysis and cluster analysis reduce dimensionality/complexity and point the researcher toward those molecules contributing most to class differences. Models based in machine learning algorithms may then be built on training datasets and validated with test datasets.
Nelson Scientific Labs provides comprehensive metabolomic services, from study design all the way to interpreting and reporting your findings. Contact us today to find out how we may help you with your metabolomics project.
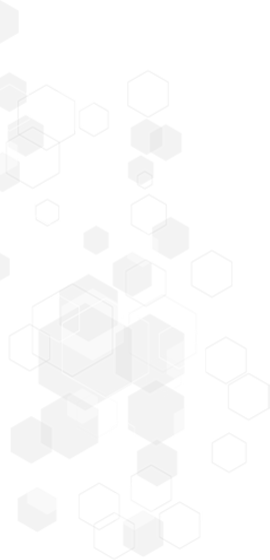